Understanding the Autocorrelation Function in Finance
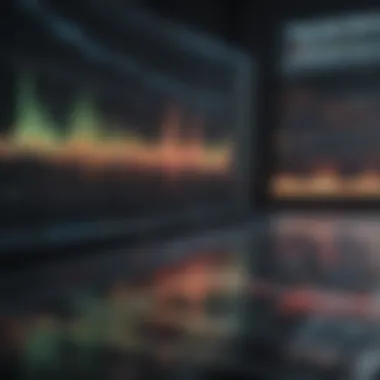
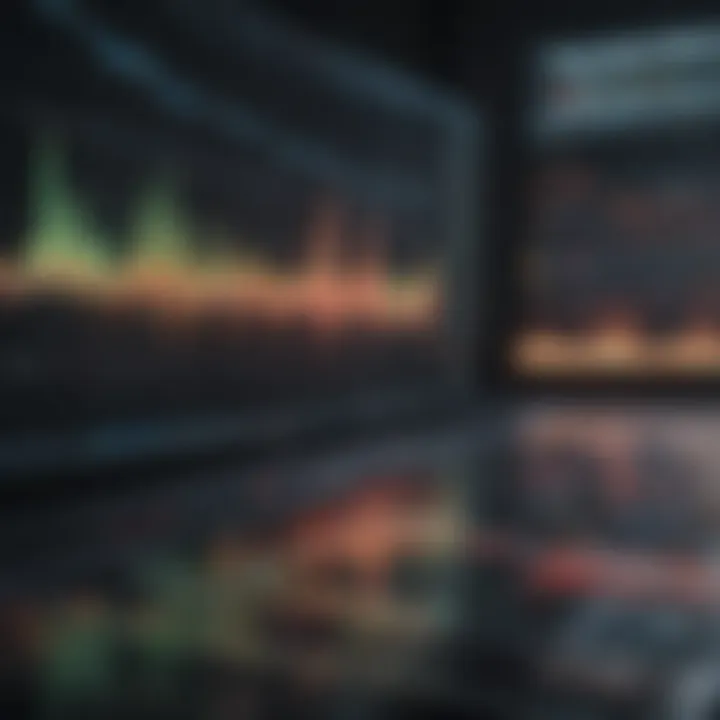
Intro
The autocorrelation function (ACF) stands as a critical tool in the toolkit of financial analysis. As financial markets grow increasingly complex, understanding the mechanisms that underpin market movements is essential. This article aims to break down the concept of autocorrelation, its significance in time series analysis, and how it impacts investment strategies. We will explore the mathematical basis of ACF, its applications, and common pitfalls encountered by financial analysts when interpreting autocorrelation data.
In the following sections, readers will delve into relevant investment terminology and gain a solid grounding in key concepts. This foundation will support a comprehensive understanding of how ACF operates in financial contexts. The discussion will not only be beneficial to novice investors but also to seasoned professionals seeking to refine their analytical skills.
Equipped with this knowledge, investors will be better positioned to leverage ACF in making informed predictions and strategic decisions in volatile markets.
Prelude to Autocorrelation Function
The autocorrelation function (ACF) is a cornerstone in the field of time series analysis, particularly in finance. Its significance cannot be overstated as it provides investors with tools to understand patterns over time in financial data. This section serves as an essential preface to the broader discussion in this article. The ACF measures how the values of a series relate to past values, helping to identify trends and cyclical behavior in market prices.
The importance of ACF lies in its ability to reveal underlying structures in financial time series data. For investors, recognizing these structures can aid in making informed decisions related to market predictions and investments. A thorough understanding of ACF enhances risk assessment, allowing for better positioning in volatile markets. In essence, utilizing ACF can be a pivotal strategy in enhancing performance evaluation of financial models.
Definition of Autocorrelation
Autocorrelation quantifies the degree of correlation between a time series and its past values. Formally, it is defined as the correlation of a signal with a delayed copy of itself as a function of delay. In practical terms, a high autocorrelation at a specific lag indicates a strong relationship between the present and past values.
The ACF is typically represented as a graph where the x-axis denotes the lag time and the y-axis represents the autocorrelation coefficient. This provides a visual representation of how past values influence current observations. The coefficients range from -1 to 1, where 1 signifying perfect positive correlation and -1 indicating perfect negative correlation. A value of 0 suggests no correlation.
Historical Context
The concept of autocorrelation has roots that stretch back to the early 20th century. Initially prominent in the fields of meteorology and engineering, its relevance in finance began to gain traction in the 1960s. Researchers such as Clive Granger developed methodologies to apply ACF in econometrics. Grangerβs work not only advanced statistical analysis but highlighted the necessity of such tools in economic forecasting.
Over the decades, the application of ACF has evolved with advancements in technology and algorithmic trading. In todayβs data-rich environment, the implementation of ACF has become more sophisticated. Financial analysts and economists now integrate ACF into robust models to better understand and anticipate market movements, thus shaping trading strategies and investment decisions.
Mathematical Formulation of ACF
Understanding the autocorrelation function (ACF) requires a grasp of its mathematical formulation. This section delves into the foundational aspects of ACF, elucidating the basic formula, its properties, and how to interpret ACF values. A clear comprehension of these mathematical underpinnings is essential for practical application in financial analysis.
Basic Formula
The basic formula for calculating autocorrelation is given by:
Where:
- r_k is the autocorrelation coefficient at lag k.
- X_t represents the value of the time series at time t.
- \overlineX is the mean of the time series.
- N is the number of observations.
This formula allows analysts to measure the degree to which current values of a time series relate to its past values. The summation processes the dataset, helping reveal patterns over specified intervals.
Properties of Autocorrelation Coefficients
Autocorrelation coefficients come with several important properties that dictate how they should be interpreted:
- Range of Values: The values of ACF typically range between -1 and 1. A value near 1 suggests a strong positive correlation, while a value near -1 indicates a strong negative correlation. A value around 0 suggests little to no correlation.
- Symmetry: The ACF is symmetric around lag 0. This implies that the autocorrelation at lag k is the same as at lag -k, reflecting the same correlation structure.
- Decaying Patterns: In many financial series, the ACF tends to decay gradually. This indicates that the influence of past values decreases over time. Understanding these patterns can help in model selection for forecasting.
The properties of autocorrelation coefficients provide crucial insights into the structure of time series, assisting analysts in detecting trends and seasonality.
Interpretation of ACF Values
Understanding how to interpret ACF values is vital when analyzing financial data. Here are some key points to consider:
- Positive Autocorrelation: Indicates that an increase in the value of a time series is likely to be followed by another increase. This is often seen in trending markets.
- Negative Autocorrelation: Suggests that high values are likely followed by low values, and vice versa. This can indicate a mean-reverting phenomenon common in some financial instruments.
- Significance Levels: Analysts often use significance tests (like the Ljung-Box test) to determine whether the observed autocorrelations are statistically significant or due to random chance. Non-significant ACF values (close to zero) suggest a lack of correlation and may imply that the series is stationary.
Understanding ACF values equips financial practitioners with insights into the underlying dynamics of financial time series data, allowing for better forecasting and strategic investment decisions.
By grasping the basic formula, characteristics, and interpretation of ACF values, one can approach financial analysis with a more informed perspective. Recognizing the significance of these mathematical foundations enhances the ability to apply these analytical tools effectively in real-world scenarios.
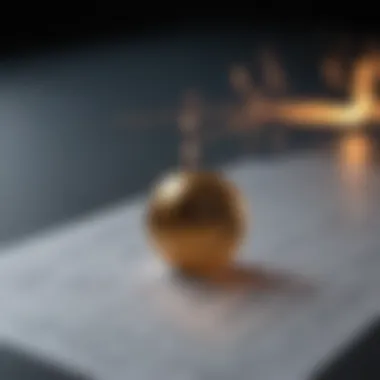
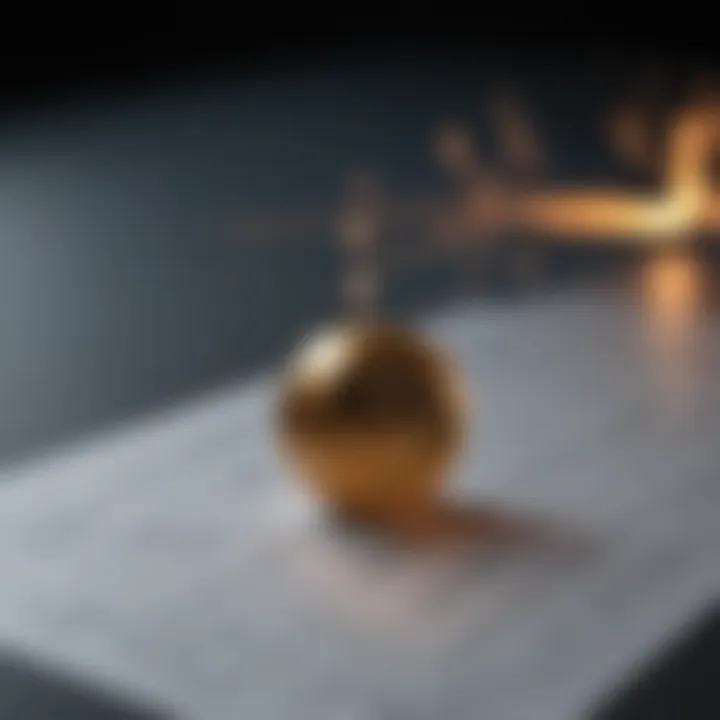
Applications of ACF in Financial Analysis
The Autocorrelation Function (ACF) plays a crucial role in financial analysis. It helps analysts and investors understand patterns in historical data that might influence future performance. By examining the correlation between observations in time series data at different lags, ACF provides valuable insights. This section explores three primary applications of ACF: time series forecasting, risk assessment and management, and performance evaluation of financial models.
Time Series Forecasting
Time series forecasting is a central application of ACF in finance. This technique uses historical data to predict future outcomes. Investors rely on this to make informed decisions about buying or selling assets. The ACF helps identify how past values in a financial series relate to future values. For example, if past stock returns show a strong correlation at lag 1, it suggests that today's return may depend significantly on yesterday's return.
Utilizing ACF in forecasting can improve the accuracy of models like ARIMA (AutoRegressive Integrated Moving Average). Understanding these correlations allows analysts to fine-tune their models, optimizing their forecasting capabilities.
Risk Assessment and Management
Risk management is another critical area where ACF provides essential functionality. By analyzing how returns behave over time, investors can identify potential risks associated with different financial instruments. The ACF assists in the detection of patterns that may help in forecasting sudden market changes.
For example, if a certain asset consistently exhibits positive autocorrelation, it may indicate that returns are becoming more predictable. Conversely, negative autocorrelation may suggest volatility. This information is vital in constructing a robust risk management strategy that balances potential returns against acceptable levels of risk.
Performance Evaluation of Financial Models
ACF can also be used as a tool for performance evaluation of financial models. After model creation, it is imperative to assess whether the modelβs predictions align with actual market data. By examining the ACF of the residuals (the differences between predicted and actual values), investors can determine whether a model captures the underlying structure of the data adequately.
If the ACF of the residuals shows significant autocorrelation, it indicates that the model may be missing essential information. In such a case, investors would need to refine the model by incorporating missing variables or adjusting parameters to improve its predictive capabilities.
"ACF helps investors track how data from different times is related, which is fundamental for making sound financial decisions."
ACF in Different Financial Contexts
The autocorrelation function (ACF) plays a vital role in understanding various financial phenomena. Its application transcends simple data analysis, providing insights that are crucial for informed decision-making. Recognizing the ACF within different financial contexts reveals its utility in interpreting complex data patterns. This understanding is indispensable for both novice and seasoned investors, as it can lead to better forecasting and strategy formulation.
Stock Market Analysis
In stock market analysis, the ACF acts as a tool for identifying trends and seasonal patterns in stock returns. Investors can assess whether past price movements influence future prices. If significant autocorrelation exists in the stock returns, it indicates that the market may be inefficient. Such inefficiencies can be exploited for potential gains.
Research has shown that positive autocorrelation can suggest upward trends, while negative autocorrelation may indicate reversals. Thus, by analyzing the ACF, traders can make more informed decisions regarding entry and exit points in their investment strategies. However, caution is advised. Over-reliance on autocorrelation metrics without considering other indicators may lead to misleading conclusions.
Interest Rate Modeling
Interest rates are pivotal in finance and the ACF assists in modeling these rates effectively. Financial analysts often study historical interest rate data to forecast future trends. ACF helps in identifying the persistence of interest rate changes over time. For instance, if a rise in interest rates has a strong autocorrelation, it may be indicative of long-term trends rather than short-term fluctuations.
This persistence can significantly influence economic policies and investment decisions. By understanding the correlations, analysts can gauge risks related to bond valuations. Hence, having a deep insight into ACF can empower stakeholders to forecast with greater accuracy.
Economic Indicators Monitoring
Economic indicators provide essential information about the health of the economy. ACF can be applied to monitor these indicators, revealing cyclical behaviors and trends. For example, unemployment rates, GDP growth, and inflation rates often exhibit temporal dependencies. By applying the ACF to these datasets, policymakers gain a clearer understanding of economic forces at play.
Utilizing ACF in this context allows for proactive measures rather than reactive responses. Strong autocorrelation in key indicators might suggest a certain economic momentum that needs to be taken into account when forming policies. Thus, ACF not only plays a role in analysis but also shapes decisions impacting large economic landscapes.
Effective utilization of the ACF provides a robust framework that supports comprehensive financial analysis across various contexts.
Challenges in Using ACF
When it comes to applying the autocorrelation function (ACF) in financial analysis, several challenges emerge. Understanding these obstacles is essential for investors and analysts. ACF, while a useful tool, is not without its complications that can affect the interpretation and decision-making process. This section will address two primary challenges: overfitting and data snooping, as well as how lagged relationships can be difficult to interpret.
Overfitting and Data Snooping
Overfitting refers to the modeling error that occurs when a statistical model describes random noise rather than the underlying relationship in the data. When using ACF, it is tempting to create overly complex models that fit historical data perfectly. This temptation can lead to the following problems:
- Misleading Results: A model that fits historical data too closely may fail to predict future outcomes accurately. Investors may become overconfident based on past performance.
- Bias in Analysis: Data snooping occurs when previously analyzed data informs model selection without considering future data. The risk here is significant. If choices are made based on mere data patterns without considering broader market fundamentals, strategies may lead to losses rather than gains.

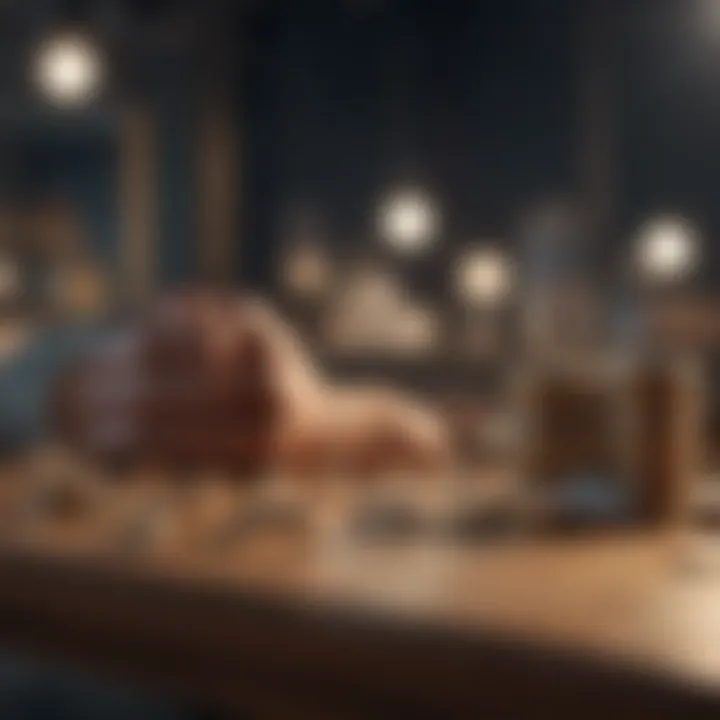
To mitigate these risks, practitioners should adopt stringent cross-validation techniques. Using separate training and test datasets can help ensure that the model generalizes well rather than fitting noise. It's crucial for an analyst to critically assess the complexity of their models to avoid these pitfalls.
"In statistics, it is essential that models can predict unseen data rather than merely explaining observed data."
Interpreting Lagged Relationships
Lagged relationships in time series data pose another challenge when using ACF in financial contexts. Understanding these relationships is vital in determining the impact of past values on current and future returns. Several considerations arise:
- Complex Dynamics: Financial markets are influenced by numerous factors at different time scales. ACF may show a high degree of autocorrelation at certain lags but fail to capture the complex dynamics of market movements.
- Lag Selection: Identifying the correct number of lags to include can be cumbersome. Too many lags can lead to confusion and overfitting, while too few may overlook critical influences.
- Misinterpretation of Results: Investors may misinterpret what high or low autocorrelation indicates about a series. For instance, positive autocorrelation can imply a trend, while negative can suggest reversals, but the context matters. Not considering exogenous variables may lead to faulty conclusions.
In summary, while ACF is a powerful analytical tool, caution is required in its application. Engaging with these challenges offers valuable insights and assists analysts in making more informed decisions.
Contrast Between ACF and Other Statistical Tools
In the domain of financial analysis, various statistical tools serve unique functions. The autocorrelation function (ACF) is particularly important for understanding relationships within time series data. However, distinguishing ACF from similar statistical tools helps clarify its unique strengths and weaknesses. Understanding these comparisons not only enhances analytical skills but also contributes to more informed decision-making.
ACF vs. PACF
The partial autocorrelation function (PACF) is often discussed alongside ACF, but they serve distinct purposes. The ACF reflects the correlation of the time series with its own lagged values. It does this for all lags in the data. In contrast, PACF concentrates on the correlation of the time series with its own lagged values while controlling for the intervening lags. This distinction is crucial when building autoregressive models.
Many analysts opt to use both functions when modeling time series data. This is because ACF helps identify the overall correlation structure, while PACF pinpoints the direct relationships that influence future observations. Therefore, in examination:
- ACF:
- PACF:
- Provides a broad view of lagged relationships.
- Useful for determining the order of a moving average model.
- Offers a clearer insight into direct influences.
- Effective for establishing the order of an autoregressive model.
Understanding these differences allows investors to select the proper tool for their analyses. It also reveals how both functions can complement each other in creating robust forecasting models.
ACF vs. Cross-Correlation Function
Cross-correlation function (CCF) gauges the relationship between two distinct time series. In contrast, ACF focuses exclusively on the relationships inherent within a single time series. This fundamental difference merits attention, particularly in comparative analyses.
The CCF can be especially valuable when examining how one variable affects another over various time lags. For example, an investor might employ CCF to evaluate whether changes in interest rates influence stock prices with a time lag. In using CCF, certain considerations arise:
- Temporal Relations: CCF captures how the relationship evolves over time, giving insight beyond immediate correlations.
- Bidirectional Analysis: CCF facilitates analyses that accommodate both series interacting, which is not possible with ACF alone.
On the other hand, the ACF is simpler for analyzing just one series. It helps highlight patterns of inertia within the same dataset.
In summation, while both ACF and CCF hold equal importance in financial analysis, they cater to distinct analytical needs. Investors must understand how and when to apply each tool effectively, thereby enabling better insight into market behaviors and trends.
Practical Examples of ACF Usage
Understanding the autocorrelation function (ACF) is invaluable in financial analysis, specifically when looking at practical examples in real-world scenarios. Practical use cases help ground theoretical knowledge and demonstrate how ACF can offer actionable insights in finance. Various sectors of finance utilize ACF effectively; therefore, it is essential for both novice and seasoned investors to grasp its significance.
ACF serves as a vital tool by revealing correlations between a financial metric and its past values. This function assists investors in identifying patterns, seasonal trends, and potential anomalies in time series data, ultimately aiding decision-making processes. The benefits of employing ACF in practical situations include enhanced accuracy in predictions, improved risk assessments, and optimal investment strategies.
Case Study: ACF in Stock Returns
Stock market returns often exhibit specific patterns influenced by numerous factors. By applying the ACF to historical stock price data, investors can evaluate how past returns are correlated with future returns. This is crucial for developing both predictive models and risk management techniques. For instance, if a particular stock has high positive autocorrelation in returns over discrete time intervals, it suggests that past performance could be indicative of future performance.
Additionally, utilizing ACF in stock returns also allows analysts to detect trends and cyclic behaviors. Seasonality may emerge as a result of recurring events influencing investor behavior, such as earnings announcements or market cycles. Recognizing these trends can guide investment decisions to maximize potential returns.
"Utilizing ACF in stock returns can guide investors in developing predictive models that consider historical performance and market trends."
It is important, however, for analysts to remain cautious. Relying solely on ACF could lead to oversights in external factors, such as market news or economic indicators that could impact stock performance. Balancing ACF analysis with other indicators creates a more solid investment strategy.
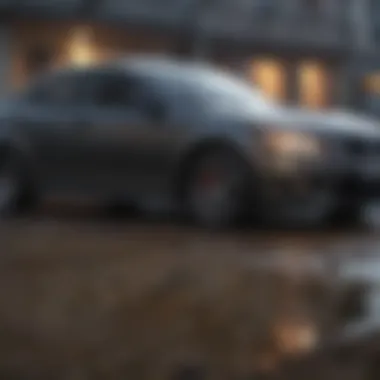
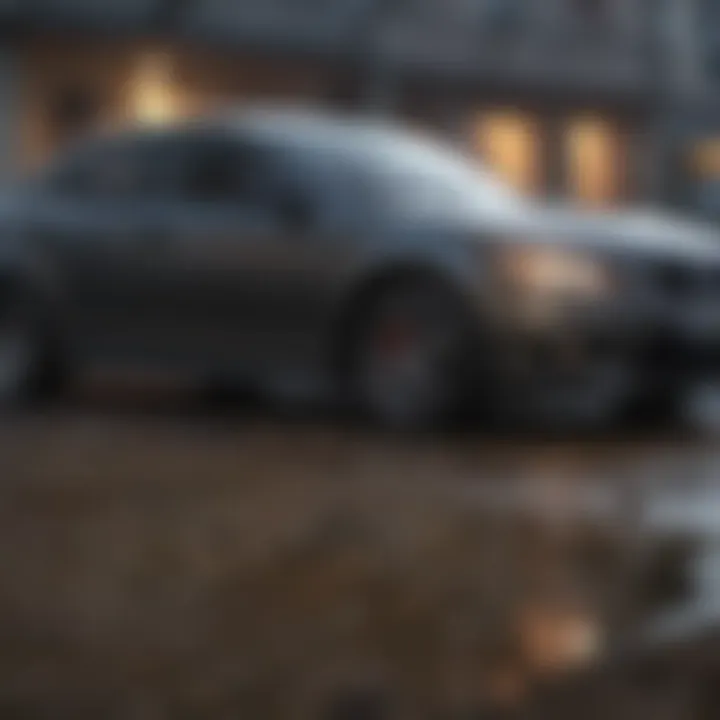
Case Study: Interest Rate Time Series
Interest rates strongly influence various elements in the economy, from consumer spending to business investments. When analyzing historical interest rates using the ACF, it becomes evident how these rates can be autocorrelated across different time frames. Investors, government entities, and economists frequently rely on ACF to assess the behavior of interest rates over time.
For example, if the ACF indicates a strong positive correlation over annual lags, it implies that fluctuations in interest rates may be influenced by earlier changes, suggesting potential predictive power. Economists can use this information when modeling economic growth or recession forecasts. Furthermore, understanding the autocorrelation in interest rates allows financial institutions to devise risk management practices better suited to volatile markets.
Assessing autocorrelation in interest rates also helps in identifying structural changes in the market. For instance, shifts in policy by central banks can play a significant role in altering interest rate behaviors. By observing these correlations and trends, policy makers and investors can respond more effectively to imminent market shifts.
In summary, practical examples of ACF usage in stock returns and interest rate analysis provide concrete insights. Investors and analysts can leverage this analytical tool to improve forecasting accuracy and gain clarity in uncertain market conditions.
Future of ACF in Finance
As we analyze the evolution of financial markets, the significance of the autocorrelation function (ACF) becomes even clearer. The future of ACF in finance is promising, as it is poised to shape decision-making processes for both investors and analysts. With the increasing complexity of market data, understanding the implications of autocorrelation provides strategic benefits that cannot be overlooked. This section discusses emerging trends and technological advances that may influence how ACF is applied in finance.
Emerging Trends in Time Series Analysis
In recent years, there has been a surge in the use of time series analysis within the financial domain. Emerging trends highlight several key aspects:
- Integration with Machine Learning: Combining ACF with machine learning models enhances predictive capacity. This integration enables investors to identify patterns and anomalies promptly.
- Shift Towards Algorithmic Trading: As the financial landscape embraces automation, ACF plays a crucial role in algorithmic trading strategies. Recognizing the historical patterns in asset price movements allows algorithms to make faster, data-driven decisions.
- Increased Focus on Real-time Data Analysis: The fast-paced nature of financial markets demands real-time analysis. ACF helps in identifying correlations between data points quickly, assisting traders in adjusting their strategies dynamically.
With these trends, it is clear that ACF will continue to evolve in its application and relevance, helping investors adapt to changing market conditions.
Technological Advances and ACF
The technological landscape in finance is changing rapidly, and this transformation impacts how ACF is utilized:
- Big Data Analytics: As big data becomes central to financial analysis, ACF helps in processing vast amounts of financial data efficiently. The ability to analyze relationships over time supports better forecasts and improved risk assessments.
- Cloud Computing: The advent of cloud technology facilitates easier access to robust analytical tools. Financial institutions can implement ACF within their systems without significant investments in infrastructure.
- Artificial Intelligence: AI continues to impact financial analysis methodologies. The interplay between AI and ACF allows for more sophisticated models that can adapt to new data and reflect ongoing market dynamics.
"Technological advances are reshaping the financial analysis landscape, and ACF remains a key component in this change."
In summary, the future of ACF in finance is intricately tied to emerging trends and technological advancements. Financial analysts and investors must pay close attention to these developments to leverage ACF for better decision-making and risk management.
End
The conclusion serves as a pivotal section in framing the insights gathered throughout the article. It reinforces the key elements discussed, allowing readers to reflect on their newly acquired understanding of the autocorrelation function (ACF) in financial analysis. This section captures the essence of why ACF is significant and how it can impact financial decision-making.
One major element of this conclusion is summarizing the various applications of ACF in the real world, particularly its role in time series forecasting, risk assessment, and evaluating financial models. These applications show how ACF can serve as a reliable tool for understanding trends and making predictions in financial markets.
Benefits of Integrating ACF in Financial Analysis:
- Enhances forecasting accuracy for time series data.
- Aids in identifying patterns that may influence asset prices.
- Provides insights to improve model selection and effectiveness.
However, the conclusion also highlights considerations practitioners should bear in mind while working with ACF. For instance, it is crucial to avoid overfitting and data snooping pitfalls. ACF should not be taken in isolation; it needs to be part of a broader analytical approach.
Importantly, the implications of ACF are far-reaching. Practitioners who grasp the intricacies of ACF can utilize it to refine their investment strategies. It empowers investors to make informed decisions based on historical patterns and correlations, potentially leading to better investment outcomes.
"Understanding ACF is not just an academic exercise; it is a practical skill that can significantly enhance the decision-making process in finance."
Overall, this concluding section summarizes the integral role of ACF in understanding financial data, highlighting its relevance and applicability to both novice and seasoned investors.
Summary of Key Points
In this section, we will encapsulate the fundamental ideas regarding autocorrelation that have been articulated throughout the article.
- Definition of Autocorrelation: ACF is a tool that quantifies the relationship of a variable with its past values.
- Mathematical Underpinning: The formula and properties reflect its utility in time series analysis.
- Applications: ACF proves essential in forecasting, risk management, and performance analysis.
- Variability Across Contexts: Its importance varies from stock market analyses to interest rate modeling.
- Challenges: There are significant risks of overfitting and misinterpretation.
- Contrasts with Other Tools: Understanding its relationship with PACF and Cross-Correlation Function fosters better analytical comprehension.
- Future Directions: Emerging trends indicate the need for advanced methodologies and technology to harness ACF effectively.
Implications for Financial Practitioners
For financial practitioners, the insights offered by ACF can drastically alter one's approach to market analysis.
- Enhanced Predictive Power: By understanding autocorrelations, professionals can make better predictions about future price movements.
- Informed Risk Management: Knowledge of past dependencies assists in recognizing potential risks in asset valuation.
- Strategic Investment Decisions: Investors equipped with ACF insights can refine their strategies, possibly resulting in higher returns.
- Decision Support: ACF can serve as a foundation for building complex models, aiding in making data-driven decisions.
- Adaptation to Change: As financial environments evolve, practitioners who utilize ACF can adjust their strategies to remain competitive.
In summary, ACF is not merely a technical concept but a practical tool that enhances financial analysis, equipping practitioners with the insights needed to navigate complex financial landscapes.