Exploring AI Applications in Finance
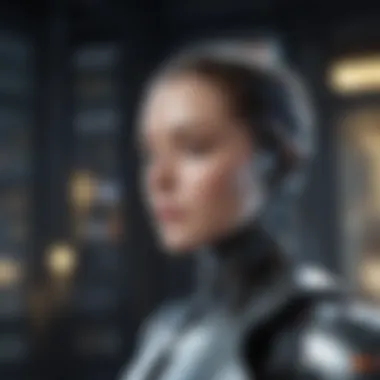
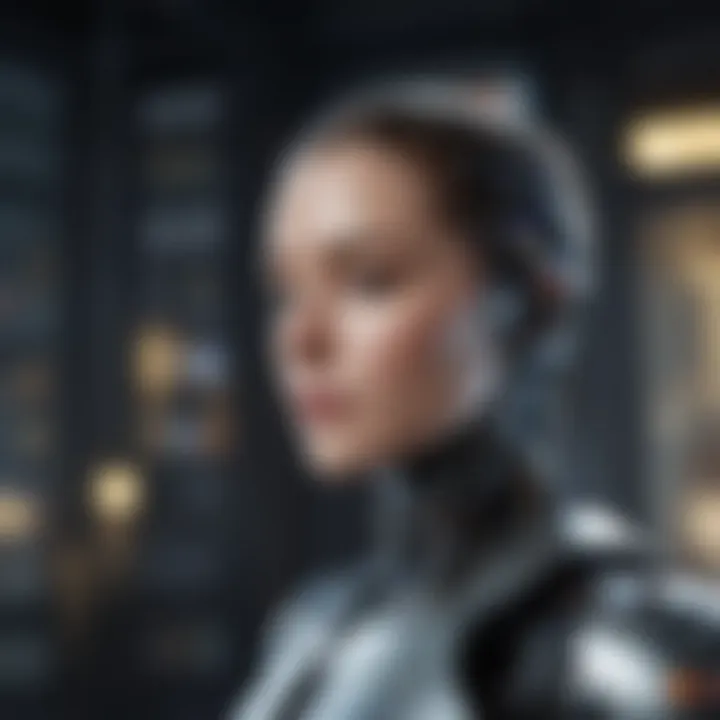
Intro
Artificial intelligence is not just a buzzword today; it's rapidly turning into a cornerstone for many sectors, and finance is no exception. The ways in which AI intertwines with financial operations are both diverse and crucial. In essence, AI can be the key to unlocking smarter investment strategies, refining risk assessment processes, and fostering more effective customer interactions within finance.
Understanding the dynamics of AI is essential in grasping its implications in finance. With this, both novice and seasoned investors will benefit significantly. The following sections will break down vital investment phrases, ensure clarity on terminology, and facilitate a better grasp of the broader themes within the field of finance and AI.
Essential Investment Terms
Overview of common investment terminology
This section aims to streamline the maze of terms often encountered in the financial landscape. By familiarizing oneself with the lingo, investors can make more informed decisions and navigate the complexities of AI-infused financial environments with greater ease.
Importance of understanding these terms
Diving into finance without a grasp of its basic terms can feel like stepping into the deep end without knowing how to swim. Knowing the terms not only helps in understanding reports and forecasts, but it also imbues confidence when discussing strategies with peers or advisors. Familiarity with these words fosters clearer communication, ultimately leading to better decision-making.
Detailed Glossary
Here's a distilled A-Z list of essential financial terms along with concise definitions that can enhance your understanding:
- Asset: Any resource owned by an entity that is expected to provide future economic benefits.
- Liquidity: Refers to how easily an asset can be converted into cash without affecting its market price.
- Portfolio: A collection of financial investments like stocks, bonds, commodities, and other assets.
- Volatility: The degree of variation in a trading price series over time, representing the level of risk associated with an asset.
- Yield: The earnings generated from an investment over a specific period, usually expressed as a percentage of the investmentβs cost.
This glossary can serve as your handy reference as we march through the AI landscape in finance.
"In investing, what is comfortable is rarely profitable." - Robert Arnott
This highlights the need to embrace complexity, which AI can bring to the table.
By leveraging AI, investors can dissect data points quickly, uncovering insights that were historically buried under heaps of paperwork and analyses. These tools are reshaping how decisions are made by facilitating a deeper understanding of various market dynamics.
In the subsequent sections, we'll explore how AI specifically alters investment strategies, refines risk management methodologies, and enhances customer interactions, along with the ethical considerations that accompany these advancements. Stay tuned!
Prolusion to Artificial Intelligence in Finance
The realm of finance is undergoing a transformation, and at the heart of it is artificial intelligence (AI). Financial institutions are increasingly turning to AI technologies to enhance their operations, manage risks, and improve customer interactions. As we navigate through various applications of AI in finance, it becomes apparent that this technology isnβt just a passing trend; itβs a fundamental shift in how data is analyzed and utilized. Understanding this shift is crucial for both novice and seasoned investors as they position themselves in an ever-evolving market landscape.
The Definition of Artificial Intelligence
Artificial intelligence, in the simplest of terms, refers to the simulation of human intelligence in machines programmed to think and learn. It encompasses a wide range of technologies, including machine learning, natural language processing, and robotics. In finance, AI is harnessed to sift through vast amounts of data and identify patterns that would be impossible for a human to decode within a reasonable time frame. The implication here is that decision-making in investment, risk management, and many other financial services can be greatly optimized. For example, rather than analyzing monthsβ worth of market trends, AI can do it in seconds, providing timely insights that can sculpt investment strategies.
Historical Context of AI in Financial Services
To appreciate the present-day application of AI in finance, it is worth delving into its historical context. The roots of AI trace back to the mid-20th century, but its serious application in finance did not gain momentum until the turn of the 21st century. Initially, algorithms were developed for basic data analysis, like modeling stock prices or assessing credit risk.
However, the turning point came with the advent of big data and advancements in computing power. Financial markets began to generate an unprecedented volume of data, supporting sophisticated algorithms that could process this information in real time.
In more recent years, machine learning and deep learning have catapulted the efficiency of financial operations, ranging from fraud detection to enhancing trading strategies.
"As financial technology evolves, so too does the sophistication of the methods used to navigate itβAI stands at the forefront of this development."
Core Technologies Behind AI
Artificial Intelligence in finance isn't just a buzzword; it's a revolution backed by sophisticated technologies. Understanding these core technologies helps us grasp how AI is changing the financial landscape. The importance lies in their ability to analyze vast amounts of data, automate processes, and enhance decision-making in ways previously unimaginable.
Machine Learning Explained
Machine learning is like teaching a child to recognize patterns through experience. In finance, it's the core of predictive analytics. Rather than explicitly program instructions, we feed algorithms historical data and let them identify trends and make forecasts. The benefits of machine learning in finance are quite staggering. For starters, it can enhance risk assessment by predicting credit defaults based on past behaviors. Algorithms can also streamline trading strategies by analyzing real-time market data, thus allowing traders to capitalize on fleeting opportunities.
To better illustrate, think of machine learning as a personal trainer who learns your strengths and weaknesses over time. Just as a trainer adapts workouts to help clients improve, machine learning adjusts its strategies based on the continuous influx of financial data.
Natural Language Processing in Finance
Natural Language Processing (NLP) is where linguistics meets intelligence. Imagine being able to sift through mountains of unstructured data, such as news articles, earnings calls, and social media chatter. NLP does just that. It not only interprets text but also derives sentiment and context. This capability opens up new avenues for investment decisions. For example, a financial analyst can gauge market sentiment regarding a company by analyzing Twitter posts and news headlines, providing invaluable insight that numerical data alone might miss.
Moreover, financial institutions use NLP to improve customer service through virtual assistants. Imagine asking your bank a question in plain English, and it promptly provides a detailed answer. That's the power of NLP, making communication more intuitive and efficient.
Robotic Process Automation Overview
Robotic Process Automation (RPA) serves as a backbone for operational efficiencies within financial services. Picture an assembly line of a factory; RPA automates repetitive tasks just like machines streamline production. It tackles various high-volume, rules-based tasks such as data entry, reconciliation, and report generation. The benefits are clear: reducing human error, improving accuracy, and freeing up human resources for more strategic roles.
For instance, a financial institution might implement RPA to automatically gather compliance documentation, allowing compliance officers to focus on more complex regulatory tasks.
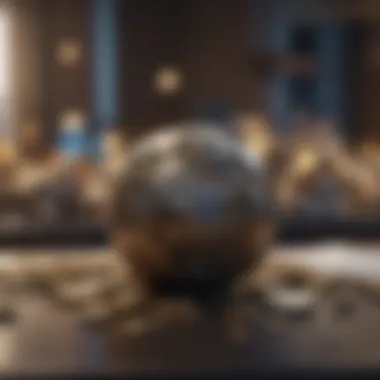
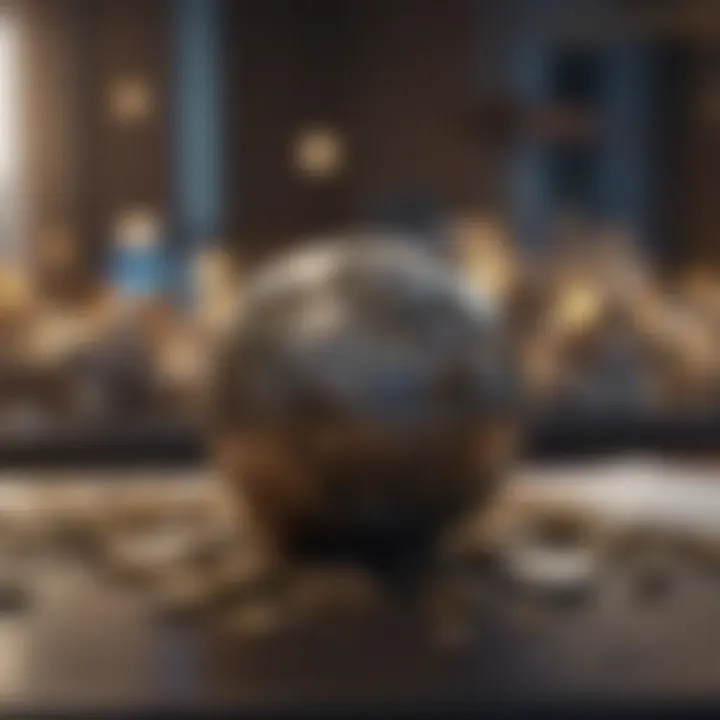
"AI applications in finance can drastically improve operations, ensuring that mundane tasks do not bog down teams that focus on strategizing and innovating."
Investment Strategies Enhanced by AI
The intersection of artificial intelligence and investment strategies marks a significant evolution in financial decision-making. Investors today face an avalanche of data and market signals, making it indispensable to leverage advanced tools that can sift through this noise. AI, armed with analytical prowess, provides strategies that not only aid in decision-making but also enhance predictability in a realm where uncertainty is prevalent.
Utilizing AI in investment strategies brings forth several advantages:
- Data Processing: AI algorithms can analyze vast amounts of historical and real-time data far quicker than any human. This efficiency enables timely decisions based on market dynamics.
- Personalization: AI-driven solutions can tailor investment recommendations according to an individualβs risk appetite and financial goals. This assures that strategies are not one-size-fits-all but rather customized to the investorβs unique situation.
- Risk Mitigation: With the use of predictive models, potential financial pitfalls can be identified early, allowing investors to recalibrate their strategies.
Given its multifaceted capabilities, AI has become an invaluable resource in shaping modern investment strategies.
Algorithmic Trading: A Deeper Look
Algorithmic trading is a prime application of AI in the investment landscape. By utilizing complex mathematical models and algorithms, traders can place orders at speeds and volumes that human traders could never match. This trading style is particularly beneficial since it allows for high-frequency trading and systematic execution of trades based on predetermined criteria, thereby minimizing emotional influences that can often lead to poor decisions.
These algorithms can also analyze market trends and monitor stock prices in real-time, allowing traders to capitalize on opportunities that require swift action. It is not only about speed; the precision with which these trades can be executed often results in better pricing.
Moreover, algorithmic trading has the potential to reduce transaction costs significantly, offering a major edge in today's competitive financial markets.
Portfolio Management Optimization
AI-powered portfolio management involves refining asset allocation to optimize returns while managing risk. Machine learning algorithms can evaluate an array of variables and forecast future asset performance, allowing managers to make informed choices about which assets to increase or decrease in weight.
The advantages of using AI for portfolio management include:
- Dynamic Adjustments: Unlike traditional methods that may rely on quarterly reviews, AI can adjust portfolios in real-time based on fluctuations in the market.
- Risk Optimization: AI tools can simulate various scenarios and estimate potential risks associated with specific assets or investment strategies, ensure portfolios are well-balanced according to the investorβs risk tolerance.
- Cost Efficiency: Through automation, AI can significantly reduce the auxiliary costs associated with managing a portfolio, making sophisticated strategies accessible to a wider range of investors.
Sentiment Analysis for Investment Decisions
Sentiment analysis has gained traction as a powerful tool for guiding investment decisions. By harnessing natural language processing, AI can evaluate public sentiment and investor mood from various sources, such as social media, news articles, and financial reports. This data provides a pulse on market sentiment that is invaluable in forecasting stock prices.
Investors can utilize sentiment analysis to:
- Understand Market Trends: By gauging the general sentiment around specific stocks or sectors, investors can make predictions about future price movements.
- React to News: AI can quickly analyze how announcements or market events impact sentiment; this can be crucial for prompt decision-making.
- Alternative Data Sources: Traditional metrics (like earnings reports) can't always tell the full story. Sentiment analysis offers additional layers of data that can lead to more nuanced insights.
In summarizing the impact of AI on investment strategies, it's clear that these tools not only facilitate better decision-making but also democratize access to sophisticated investment methodologies. Investors, whether novice or seasoned, stand to benefit from understanding and incorporating these innovative AI-driven strategies into their financial decisions.
Risk Management Techniques Utilizing AI
In todayβs fast-paced financial environment, effective risk management is more critical than ever, especially considering the increasing complexity and volatility of markets. Artificial Intelligence (AI) brings forth advanced methodologies that assist in identifying, assessing, and mitigating risks. The deployment of AI in risk management offers various advantages that can significantly improve the decision-making processes of financial institutions.
AI-powered risk management techniques harness vast swathes of data, providing deeper insights that traditional methodologies often overlook. This enables firms to predict potential downturns and adjust their strategies accordingly, and it enhances compliance with regulatory requirements. Moreover, by automating routine tasks, firms can allocate their resources more efficiently, focusing on strategy rather than merely execution.
Predictive Analytics in Risk Assessment
Predictive analytics is a cornerstone of modern risk assessment in finance. It utilizes historical data, statistical algorithms, and machine learning techniques to identify the likelihood of future outcomes based on current and past data.
By analyzing patterns and trends within financial data, predictive models can forecast potential risks, whether in investments, lending, or market fluctuations. For instance, banks can use predictive analytics to assess the creditworthiness of loan applicants more accurately by scrutinizing their past financial behavior and external data such as market trends and economic factors. This not only improves loan performance but also minimizes default risks.
Key benefits of predictive analytics include:
- Enhanced accuracy in risk assessment
- Timely identification of emerging risks
- Resource optimization through predictive insights
Fraud Detection via AI
The financial sector has long been a target for fraud, and traditional methods of detection have often struggled to keep up with increasingly sophisticated tactics employed by fraudsters. AI systems, particularly those using machine learning, can analyze patterns in transactions to spot anomalies that indicate potential fraud.
For example, companies like PayPal and Mastercard use AI algorithms to monitor transactions in real time, flagging any that deviate from typical behavior. By employing these AI-driven fraud detection systems, businesses can significantly reduce false positives and accurately identify fraudulent activities, thereby protecting both their assets and their clients' trust.
"Using AI for fraud detection is not just about speed; itβs about accuracy. The quicker you can identify a fraudulent transaction, the better you safeguard your enterprise.β
Stress Testing Financial Models
Stress testing is a critical process that involves simulating various adverse economic conditions to assess how financial institutions might perform under pressure. AI enhances this process by allowing firms to conduct more comprehensive and frequent tests than traditional methods would permit.
By utilizing AI, financial firms can analyze multiple factors simultaneously and create more realistic stress scenarios. For example, institutions can evaluate how a sudden economic downturn, combined with rising interest rates and shifting regulatory landscapes, could impact their portfolio performance.
Benefits of AI in stress testing include:
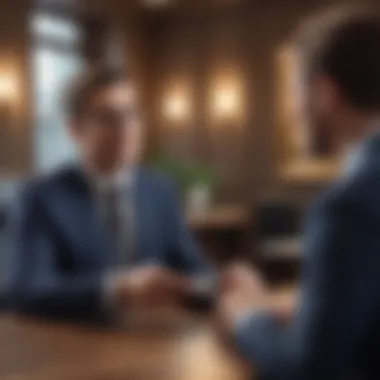
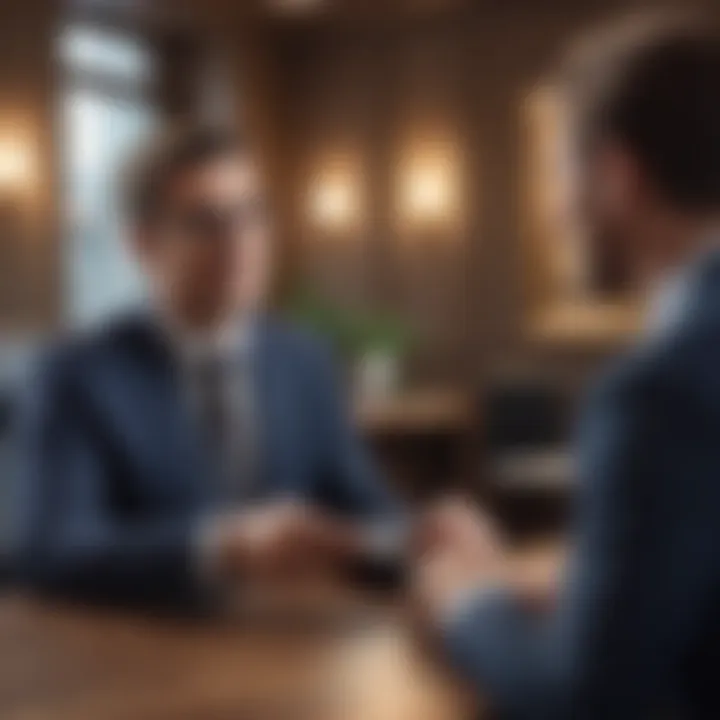
- Increased frequency of tests without resource drain
- Development of complex scenarios that reflect real-world uncertainties
- Better preparedness for unexpected events, minimizing losses
As we navigate the complexities of the finance sector, the application of AI in these risk management techniques not only helps in mitigating risks but also streamlines overall operations, making institutions more agile and responsive to market changes. By adopting these advanced technologies, financial organizations can stay one step ahead of potential threats.
AI's Role in Enhancing Customer Experience
Artificial intelligence has become a linchpin in revolutionizing how financial institutions engage with their customers. The significance of AI in enhancing customer experience cannot be overstated. From the moment a client interacts with a service provider, AI is working behind the scenes, optimizing every touchpoint. The focus here encompasses the use of chatbots, the personalization of financial products, and the streamlining of client interactions. These advancements not only foster satisfaction but also build stronger relationships and drive loyalty.
Chatbots and Virtual Assistants
Chatbots and virtual assistants are the frontline soldiers in AI's mission to improve customer service in finance. These technologies enable financial institutions to offer 24/7 assistance. Imagine a customer needing help with their account in the dead of night. They can simply message a chatbot that swiftly provides answers, alleviating frustration.
The benefits of chatbots include:
- Instant Response Times: They handle common queries swiftly, reducing wait times.
- Consistency in Answers: Chatbots maintain a high level of accuracy in their responses, ensuring customers receive the correct information every time.
- Cost Efficiency: By managing simple queries, chatbots free up human agents to tackle more complex issues, optimizing resource allocation.
However, implementing chatbots isn't without its challenges. Ensuring they understand nuanced customer inquiries can be tricky. Not everyone feels comfortable talking to a machine, so striking the right balance between automation and human touch remains crucial.
Personalization of Financial Products
In a world where one size does not fit all, personalizing financial products emerges as a game-changer. AI analyses historical data and behavioral patterns to tailor financial solutions to individual needs. For example, consider how a bank might offer customized loan options based on a customer's spending habits and credit history.
Some key aspects include:
- Targeted Offers: By analyzing user data, institutions can provide relevant product recommendations, enhancing the likelihood of customer satisfaction and conversion.
- Improved Trust: Personalization fosters a sense that a financial institution understands its clients, potentially leading to increased trust and long-term relationships.
- Enhanced Engagement: Customers are more likely to interact with tailored communications. This heightened engagement can bolster a brand's reputation.
Though personalization has its merits, challenges surrounding privacy and data protection must be seriously considered. Striking the right balance between relevant offers and respecting customer privacy is vital.
Streamlining Client Interactions
Streamlining client interactions is another area where AI shines brightly. This encompasses everything from simplifying the onboarding process to providing seamless transaction experiences. Automation tools can handle data entry, regulatory compliance tasks, and even the gathering of customer feedback, all while minimizing errors.
Key advantages of streamlining include:
- Efficiency Gains: Clients appreciate a quicker onboarding experienceβwhat was once a cumbersome process can now be completed in minutes.
- Frictionless Transactions: AI makes it easier for customers to execute transactions, reducing the chances of abandonment due to frustration.
- Informed Client Interactions: Financial advisors equipped with AI tools can offer insights and recommendations tailored to each client's unique circumstances.
To wrap it up, AI plays a pivotal role in enhancing customer experience in finance, encompassing chatbots, personalized products, and streamlined interactions. As financial institutions navigate the complexities of this digital landscape, the pursuit of effective AI implementations continues. It's essential that they remain vigilant about the potential pitfalls while harnessing the undeniable benefits that AI brings to the table.
"As technology evolves, so do customer expectations. The integration of AI into finance is not merely an upgrade; itβs a transformation that caters to the modern client's needs."
This evolution is not just a trend; itβs a fundamental shift towards a more customer-centric financial landscape.
Ethical Considerations in AI Implementation
In the age of technology, the integration of artificial intelligence in finance raises numerous ethical considerations that cannot be overlooked. These issues hinge not just on how AI operates, but on the implications of its decisions, its development, and its interactions with users. As AI continues to change how financial transactions occur and how insights are drawn from data, the scrutiny surrounding these technologies increases. The significance of ethical considerations becomes evident when we discuss the real-world impact they have on individuals and society overall.
One primary concern centers on bias in algorithms. Algorithms are only as impartial as the data they are trained on. If the underlying data reflects societal biases, the resultant AI outcomes can perpetuate discrimination. For example, if a financial institution relies on historical lending data that exhibits racial or gender biases, any AI developed from it may continue these patterns, further disenfranchising marginalized groups. This critical issue needs ongoing attention to ensure fairness and equity in AI-powered finance.
The ethical implications also stretch to data privacy concerns. With AI systems sifting through vast amounts of personal financial information, thereβs an ever-present risk of misuse or mishandling of sensitive data. Individuals may find themselves at risk if their financial data is inadequately protected or exploited without their consent. Hence, financial entities must adopt stringent data protection measures, ensuring they respect user privacy while still leveraging the valuable insights AI can provide.
Lastly, transparency in AI processes must become a priority. Users and stakeholders should have a clear understanding of how AI systems make decisions. Often, these AI systems function as a 'black box,' making it difficult to ascertain how certain conclusions are reached or what data influenced them. By promoting transparency, institutions can help build trust among users, allowing them to understand the rationale behind AI-driven financial decisions. This could be achieved through clearer documentation and communication strategies that effectively demystify AI processes.
"Navigating the ethics of AI is not just an obligation; itβs the foundation of trust in financial services."
These ethical considerations lead us to a pivotal question: How can financial institutions implement AI responsibly? By recognizing and addressing bias, ensuring data privacy, and fostering transparency, they can create an ethical framework that promotes trust while harnessing the power of AI. This represents not only a way to comply with regulations but also an opportunity to differentiate themselves positively in a competitive landscape.
In summary, addressing ethical considerations in AI is crucial for not only safeguarding user interests but also fostering sustainability and fairness in financial services. As the sector grows, so too must the discussions around the ethical ramifications of AI, ensuring advancements do not come at the cost of societal values.
Challenges of AI in the Financial Sector
In the rapidly evolving landscape of finance, the integration of artificial intelligence brings with it a host of opportunities. However, the road is not paved with gold, as there are significant hurdles that financial institutions must navigate. Understanding these obstacles is vital not just for tech enthusiasts but also for investors who must reckon with the implications of these challenges. Letβs dive into three core challenges that define the AI journey in finance: integration difficulties in legacy systems, the skills gap in the workforce, and regulatory compliance issues.
Integration Difficulties in Legacy Systems
One of the initial roadblocks encountered when implementing AI in finance is the challenge of integrating new technologies into established legacy systems. These systems, often built decades ago, are like old ships set in their ways, slow to change, and resistant to new additions. While they may still function, they often lack the flexibility required to adapt to advanced AI solutions.
The crux of the issue lies in the architecture of these systems. Outdated programming languages and siloed data environments make it incredibly difficult to layer in AI functionality. For instance, a banking institution that relies on an aging mainframe may struggle to employ advanced machine learning algorithms, which typically require a more dynamic data environment.
Moreover, the costs associated with upgrading or replacing these legacy systems can be formidable. Financial firms often find themselves caught between a rock and a hard place, balancing the need for modernization against the operational upheaval that upgrading can entail. Understanding these integration challenges is crucial, as they can delay or derail otherwise promising AI initiatives.
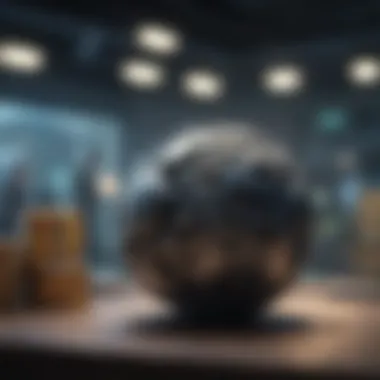
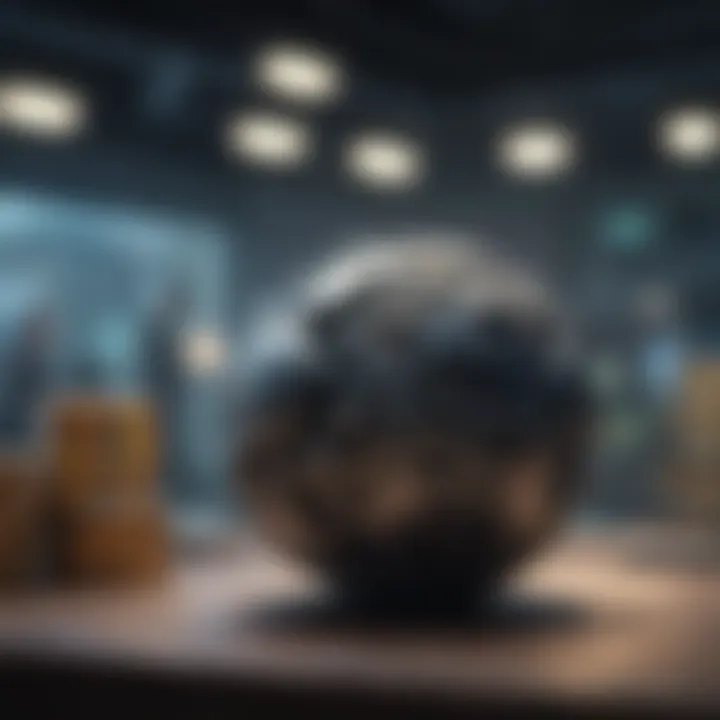
Skills Gap in the Workforce
As financial institutions strive to embrace AI technologies, they are often hindered by a skills gap in their current workforce. Many employees may possess traditional financial expertise but lack the technical acumen needed to leverage AI tools effectively. This gap creates a scenario where promising technologies may go underutilized or employed ineffectively.
Key factors contributing to the skills gap include:
- Limited Training Opportunities: Many organizations do not provide adequate training programs focused on AI applications.
- Fear of Automation: There is often anxiety among employees that AI will replace their jobs, causing resistance to learning new skills.
- Rapid Evolution of Technology: The quick pace of AI development means that training can often feel outdated almost as soon as it happens.
ToAddress this gap, organizations might consider investing in continuous professional development, offering workshops, and creating a workplace culture that embraces lifelong learning.
Regulatory Compliance Issues
Another pressing challenge lies in the realm of regulatory compliance. The financial sector is one of the most heavily regulated industries, and the introduction of AI adds another layer of complexity to compliance protocols. Regulators are still grappling with understanding and governing AI technologies, which often leads to uncertainty in compliance requirements.
"AI doesnβt mean bypassing regulations; rather, it requires us to rethink how we approach compliance in an AI-driven world."
However, non-compliance can carry severe repercussions, including hefty fines and damage to reputation. Financial institutions must maneuver through a labyrinth of laws and regulations, ensuring their AI implementations do not inadvertently lead to breaches.
Challenges include:
- Understanding the Regulatory Landscape: The continuously evolving rules necessitate that firms remain vigilant and proactive.
- Data Governance: Proper management of data used in AI, particularly concerning personal information, is a non-negotiable in compliance.
- Audit Trails: AI systems should provide adequate monitoring and traceability to ensure accountability.
In summary, while AI holds transformative potential for the financial sector, it is not without its stumbling blocks. Addressing integration difficulties, the skills gap in the workforce, and regulatory compliance issues will be crucial for firms looking to ride the AI wave successfully.
Future Prospects of AI in Finance
The future of AI in finance is not just an afterthought; itβs quickly becoming a cornerstone of how financial services operate. As AI technologies continue to evolve, the implications for finance are vast and complex. This section will shed light on several important elements, benefits, and considerations surrounding this ever-evolving landscape.
Emerging Trends and Innovations
New trends in AI are shaping the finance industry like never before. Notably, the rise of quantum computing is beginning to make waves. Companies are starting to explore how quantum algorithms can handle calculations at speeds a classical computer simply can't. For instance:
- Enhanced Data Processing: Utilizing quantum mechanics to speed up data processing can unlock new levels of insight for traders and portfolio managers.
- Risk Modeling: Advanced simulations that currently take days could be reduced to mere hours or even minutes.
Another noteworthy trend is the surge of AI-driven robo-advisors. These platforms use algorithms to provide personalized financial advice at a fraction of traditional costs.
- Cost Efficiency: They optimize investment strategies based on user profiles and market conditions, broadening access to financial services for the average consumer.
- Integration with Social Media: This opens doors for new data sources, where opinions and trends can lead to better investment strategies.
Moreover, clients are increasingly demanding tailored and intuitive product offerings. Innovations in machine learning enable firms to analyze massive datasets, leading to products that suit individual needs more closely than ever before.
Potential Disruptions to Traditional Models
AI doesn't just enhance existing practices; it has the potential to flip the script entirely. Traditional banking models are already feeling the tremors of AI adoption.
- Disintermediation: Peer-to-peer lending platforms and blockchain technology are examples of how direct transactions can bypass banks, cutting costs and increasing accessibility.
- Market Democratization: This shift gives retail investors access to tools previously only available to institutional investors, leveling the playing field.
The rise of decentralized finance (DeFi) could be another significant disruptor. Unregulated lending platforms enable transactions without traditional intermediaries, posing questions about risk and accountability that weren't present before.
The Evolving Regulatory Landscape
As AI gains traction in finance, regulatory bodies face growing challenges to ensure consumer protection while fostering innovation. Laws must evolve to keep pace with technology, becoming less reactive and more proactive.
- Transparency: Regulators will need to insist on clear explanations of how AI algorithms make decisions, addressing the opacity of machine learning models.
- Consumer Rights: Ensuring clients understand how their data is used becomes crucial, particularly concerning privacy issues and algorithmic bias.
- Standardization: A need for standardized regulations across jurisdictions may arise to harmonize guidelines, which could ultimately facilitate greater adoption across borders.
While navigating this landscape may seem daunting, understanding it is vital for investors and financial professionals alike.
"The very nature of finance will be redefined by AI, but its successful integration depends upon adept regulation and ethical considerations."
In essence, the future prospects of AI in finance teem with both opportunities and hurdles. As technologies and their implications mature, stakeholders must stay informed and adapt to these transformative changes.
Finale
In the realm of finance, the integration of artificial intelligence (AI) has marked a significant turning point. The conclusions drawn from this article encapsulate the sweeping changes AI has brought about in investment strategies, risk management, and customer engagement. Itβs clear that AI is not a passing fad but rather a fundamental shift in how financial service operations are conducted. This new technology supports decision-making in a rapidly evolving landscape, offering clarity amidst the chaos of big data.
Summarizing the Impact of AI
The implementation of AI technologies has fundamentally transformed several aspects of finance. Here are some key impacts:
- Enhanced Decision-Making: AI algorithms can process vast amounts of data far quicker than any human analyst. This speed allows for more timely and informed decisions regarding investments and risk assessment.
- Improved Customer Interactions: Tools like chatbots and automated customer service platforms have streamlined client communications, making it easier for customers to access information and services. This innovation not only saves time but also significantly enhances user experience.
- Risk Mitigation: AI's predictive capabilities help in forecasting potential financial pitfalls. By utilizing machine learning, financial institutions can better identify risky behaviors, significantly reducing instances of fraud or poor investment decisions.
Overall, the value AI adds to finance lies not just in efficiency but in accuracy.
The Road Ahead for Financial Services
Looking forward, the trajectory of AI in the financial services sector appears bright yet challenging. Several factors will shape the future:
- Continuous Evolution of Technology: As machine learning and automation become more sophisticated, their capacity to analyze and exploit financial data will only improve. The finance industry must remain adaptable to harness these advancements.
- Regulatory Frameworks: With the proliferation of AI, regulatory bodies are likely to develop more stringent guidelines to govern their use. Financial services must navigate this evolving landscape without stymieing innovation.
- Ethical Considerations: As AIβs role expands, ensuring fairness and accountability in its application will be paramount. Developing algorithms that are free from bias will become not just an option but a necessity for long-term sustainability.